Quick links to the information:
Introduction & Case description
Sentiments in Malay and English
This project delves into the captivating world of human emotions, exploring how they manifest in both written and spoken languages. Here, we analyze sentiments expressed in two vibrant tongues: Malay and English, weaving through the intricacies of text and audio.
Technical Details:
- Data: A curated dataset of text and audio recordings in both Malay and English covering various domains (e.g., social media, news, interviews).
Text Analysis:
- Lexical analysis: Employing sentiment lexicons and rule-based approaches to identify sentiment-carrying words and phrases.
- Machine Learning: Building and training classifiers using supervised learning techniques like Naive Bayes, Support Vector Machines, or Recurrent Neural Networks (RNNs) to automatically recognize sentiment in text.
Audio Analysis:
- Feature extraction: Extracting acoustic features like pitch, energy, and mel-frequency cepstral coefficients (MFCCs) to capture emotional cues in speech.
- Machine Learning: Similar to text analysis, training classifiers using audio features to classify spoken sentiment.
Read Less
Read More
0
Ai Developers
0
Data Scientist
0
Language Professionals
The solution & Technology
Data
A curated dataset of text and audio recordings in both Malay and English covering various domains (e.g., social media, news, interviews).
Text Analysis
- Lexical analysis: Employing sentiment lexicons and rule-based approaches to identify sentiment-carrying words and phrases.
- Machine Learning: Building and training classifiers using supervised learning techniques like Naive Bayes, Support Vector Machines, or Recurrent Neural Networks (RNNs) to automatically recognize sentiment in text.
Audio Analysis
- Feature extraction: Extracting acoustic features like pitch, energy, and mel-frequency cepstral coefficients (MFCCs) to capture emotional cues in speech.
- Machine Learning: Similar to text analysis, training classifiers using audio features to classify spoken sentiment.
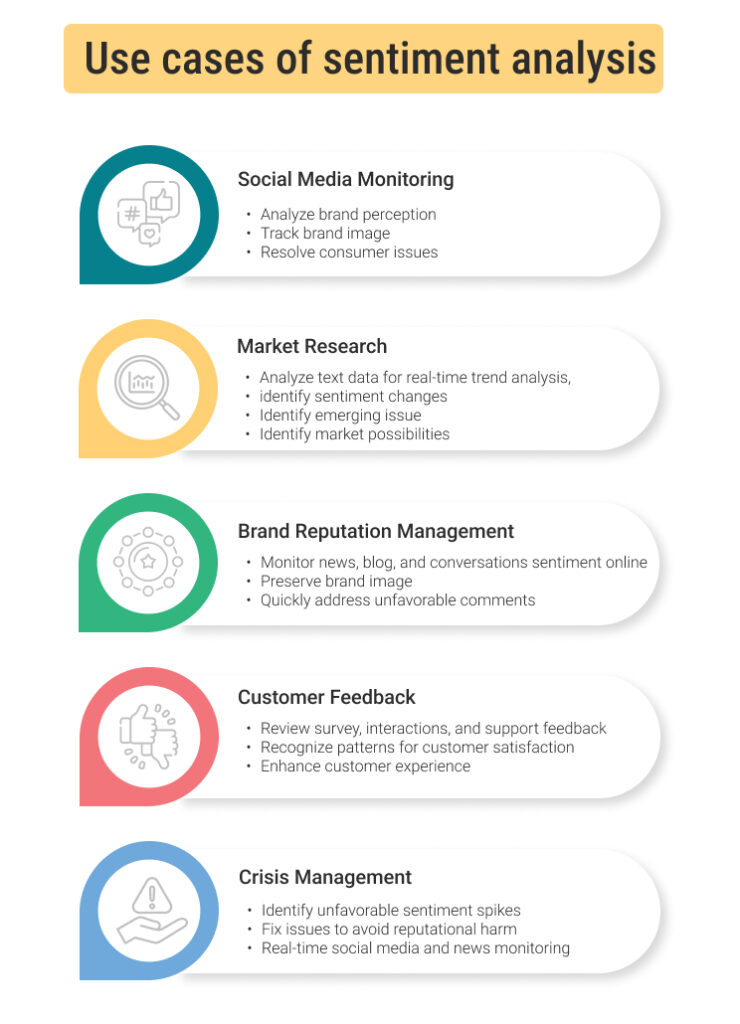
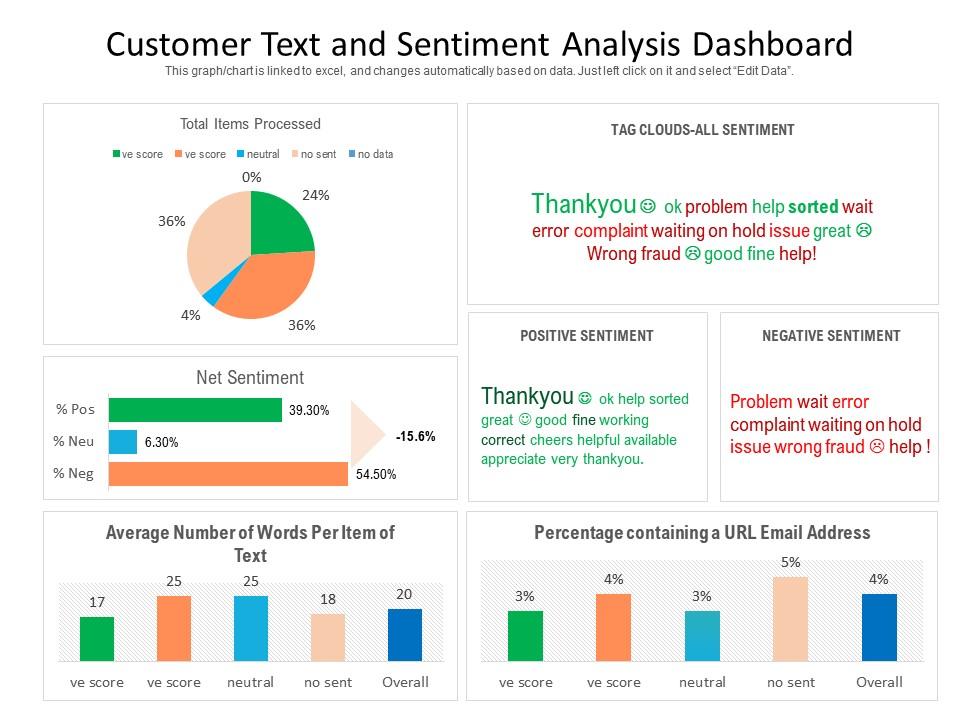
Result Driven
- Challenges and Solutions:
- Language nuances: Adapting sentiment analysis techniques to handle the specificities of Malay language, including informal slang and cultural references.
- Audio complexity: Addressing background noise, speaker variations, and non-verbal cues to accurately interpret spoken sentiment.
- Outcomes:
- Identifying positive, negative, and neutral sentiment trends in Malay and English text and audio data.
- Gaining insights into public opinion, customer feedback, and emotional responses in various contexts.
- Potential for applications in social media analysis, market research, and sentiment-aware AI systems.
"What impressed us the most was Leprofile's collaborative approach. They took the time to understand our unique needs and challenges, ensuring that the solution was tailored to our specific requirements. The team's professionalism, dedication, and innovative thinking were evident throughout the project."
Adenan, Project Director, TNB Careline